Artichoke deep learning detection network for site-specific agrochemicals UAS spraying
Oct 1, 2023ยท,,,
,,ยท
0 min read
Alberto Sassu
Jacopo Motta
Alessandro Deidda
Luca Ghiani

Alberto Carlevaro
Giovanni Garibotto
Filippo Gambella
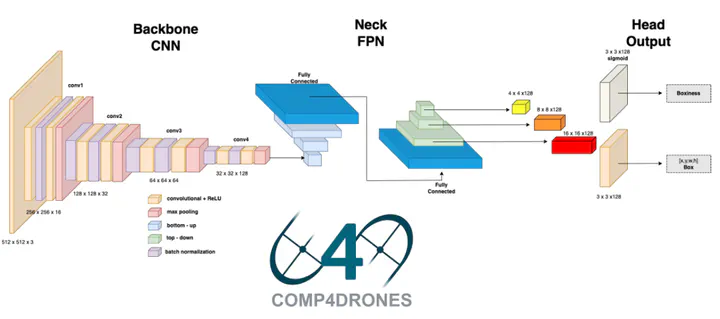
Abstract
Input optimization is a distinguishing characteristic of Precision Agriculture approaches, helping reduce the environmental impact and costs and increase vegetable production quality. Thanks to the high automation evolution of Unmanned Aerial Systems (UAS), a new approach derived from their combination with Deep Learning techniques is leading to significant improvements in agricultural management practices. The study aims at artichoke plants detection and georeferencing as a first step for an on-the-fly, real time, UAS spraying system, and use the gathered information to monitor crop development through a multi-temporal approach. A commercial UAS, equipped with an RGB sensor, acquired images of the artichoke field located in Sardinia (Italy) during the 2021โ2022 season in different crop growth stages. The FPN (Feature Pyramid Network), trained and compared with the YOLOv5 (You Only Look Once) network, showed a high detection level with an average F1 score of around 90%, and satisfactory off-line performances on the Nvidia Jetson Nano board. YOLOv5 achieved the best overall result. The FPN recorded a lower recall, which is desirable to achieve a minimum number of detection errors and limit the leakage of agrochemicals on false-positive targets. The multi-temporal approach influenced detection performances, with an inverse response of precision and recall metrics. The growing index trend showed a distinct value in October, peaking at the beginning of December as expected. The proposed approach contributes to designing future automatic and reliable site-specific UAS agrochemicals application and the classification of management zones.
Type
Publication
In Computers and Electronics in Agriculture